Machine Learning
in Remote Sensing
Interested in learning how to apply machine learning to remote sensing data? Start your journey with RSPROC!
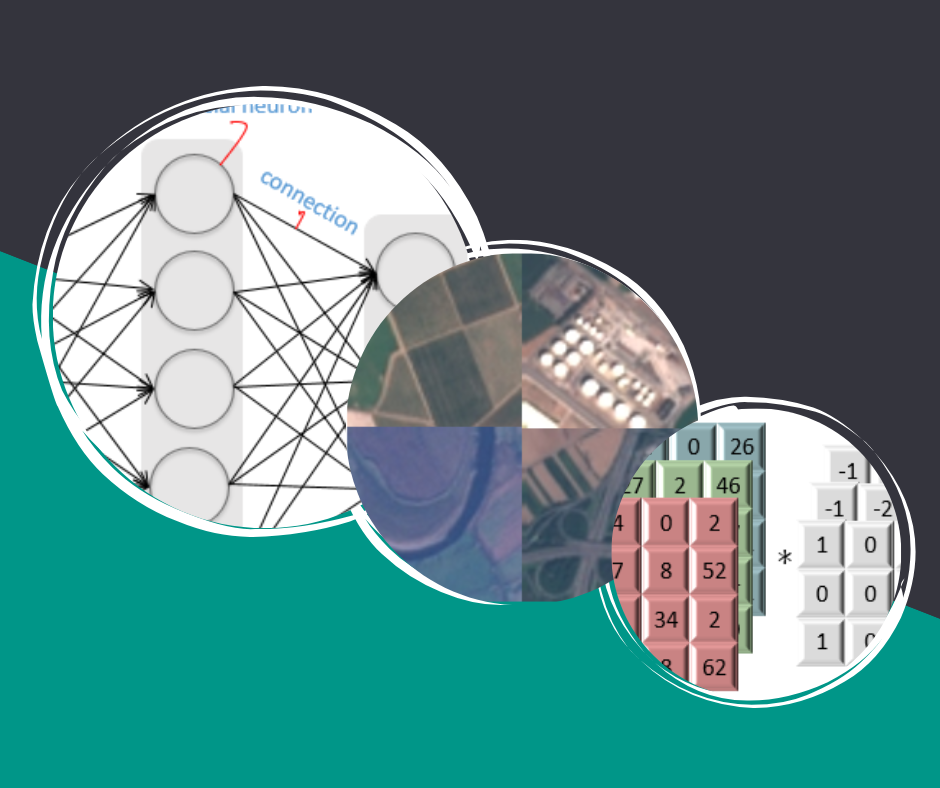
WHAT you will learn
Section 1: Foundations of Remote Sensing and Python Programming
In the first part, you will learn the foundational knowledge and skills necessary for both remote sensing and Python programming.
- Module 00: Python (Jupyter Notebook, Python Basics, Python Web Framework – Django)
- Module 01: Remote Sensing
- Module 02: Google Earth Engine (Google Colab, Google Earth Engine Python API, Machine Learning in Earth Engine)
Section 2: Methods for Learning from Remote Sensing Data
In the next part, you will explore techniques for learning from remote sensing data.
- Module 03: Machine Learning (Introduction to Machine Learning, Evaluation Metrics for Machine Learning, Creating Training Dataset for Machine Learning)
- Module 04: Parameter Estimation (Naive Bayes classifier, Maximum Likelihood, Parzen window)
- Module 05: K Nearest Neighbors
- Module 06: Principal Component Analysis
- Module 07: Support Vector Machine
- Module 08: Decision Tree (Decision Tree, Bagging, Boosting)
- Module 09: Random Forest
- Module 10: Logistic Regression
Section 3: Advanced Topics and Applications
In the final part, you will delve into advanced topics, which will enable you to acquire more specialized knowledge and skills in the field.
- Module 11: Neural Network (Artificial Neural Networks, Deep Neural Networks and Deep Learning, Convolutional Neural Networks, Transfer Learning, Data Augmentation, and more)
- Module 12: Clustering
- Module 13: Regression
- Module 14: Application
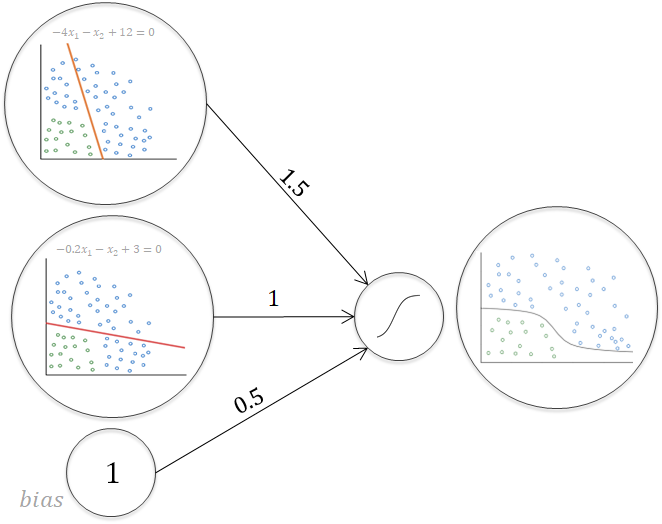
Getting Started With Neural Networks
Do you know how this curve can be obtained?
- Fundamental
- Advanced
- Python
- Remote Sensing Data
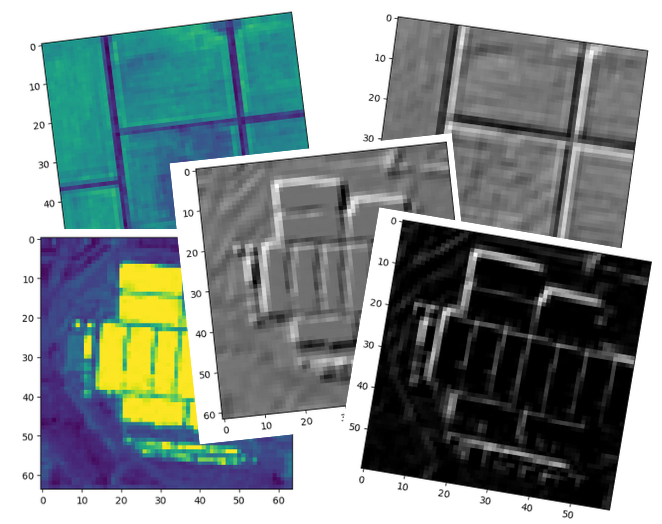
Getting Started With Deep Learning
Are you aware of the significance of convolution and convolutional layers?
- Fundamental
- Advanced
- Python
- Remote Sensing Data